ChatGPT Operator automates web tasks like booking flights, shopping, and data retrieval. While effective for basic workflows, it struggles with large-scale scraping and websites with advanced bot detection.
DeepSeek and BQL provide a more flexible solution. DeepSeek generates dynamic search queries, while BQL handles bot-resistant scraping and multi-step automation. This article compares ChatGPT Operator to the DeepSeek + BQL stack, showing how they offer greater customization and scalability.
ChatGPT Operator: What It Can and Can't Do

What ChatGPT Operator Does Well
ChatGPT Operator offers a convenient and easy way to automate online tasks. It can help with actions like browsing web pages, filling out forms, and executing workflows the way you want. Translating your natural language commands into actions makes it interact seamlessly with websites. This is especially useful for automating tasks like submitting forms, navigating web pages, or handling repetitive activities that usually need direct user input.
The tool also supports integration with external APIs, allowing it to work with structured data sources. When an API is available, ChatGPT Operator can retrieve data, process it, and adjust its actions based on the information it receives. This capability allows users to combine website interactions with external data, making it useful for tasks that involve pulling data from third-party services while automating parts of the web experience.
Where It Falls Short
ChatGPT Operator is a great basic automation option with some technical limitations. For instance, it doesn’t scrape data directly from websites, which can be tricky when working with pages that use client-side rendering or don’t provide accessible APIs. Without this ability, the tool can’t interact with what you see on the page and won’t be able to access content hidden behind JavaScript-rendered elements.
Bot detection systems present another challenge. Websites protected by services like Cloudflare or those using behavioral detection measures can block the operator’s requests. The tool does not support handling CAPTCHAs, rotating user agents, or using proxies to avoid detection. This can prevent it from accessing certain sites altogether.
Additionally, it lacks session persistence, making it tricky to carry out workflows that require a consistent logged-in state throughout different tasks. These technical limitations can reduce the operator’s efficiency when handling more intricate automation tasks involving advanced web protection and ongoing sessions.
DeepSeek + BQL: A More Flexible & Powerful Alternative
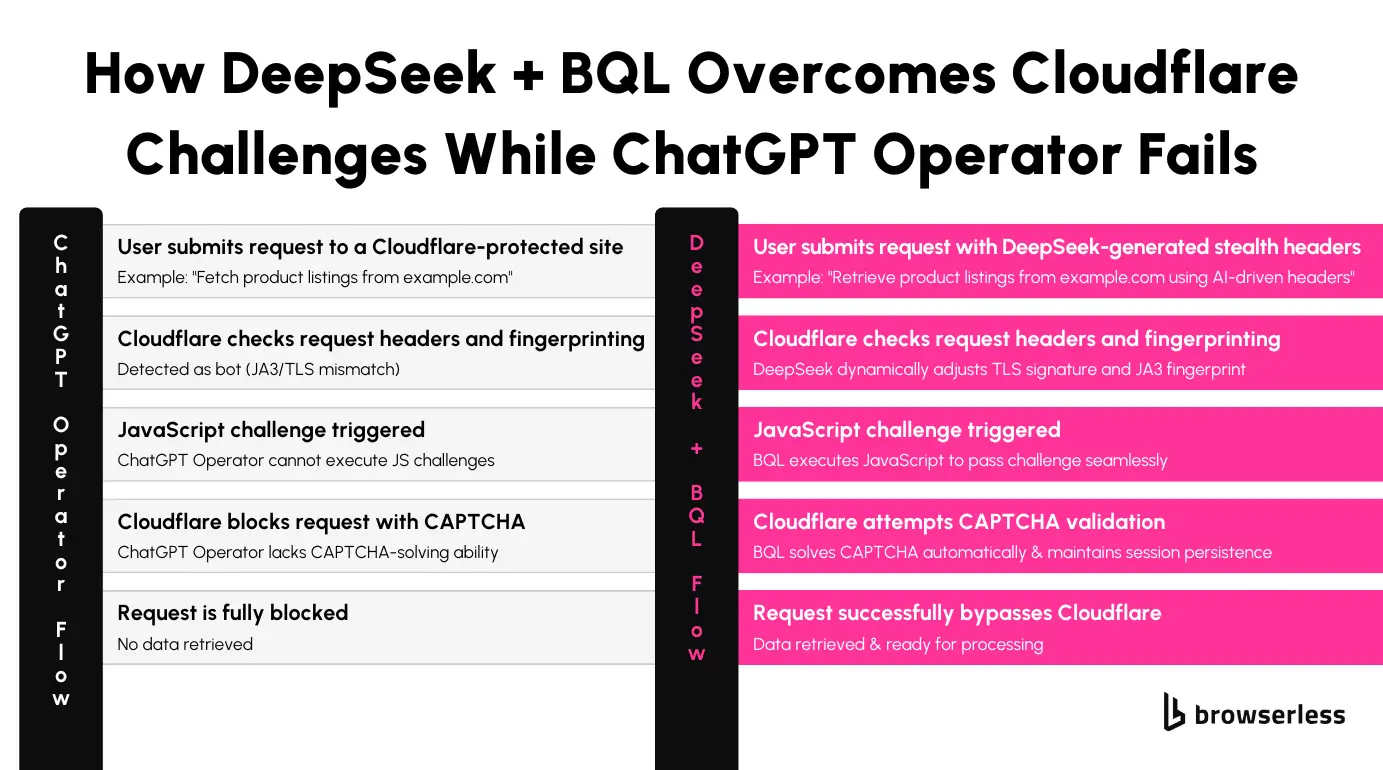
What DeepSeek Adds to the Stack
DeepSeek provides AI-driven decision-making that interprets and automates complex workflows. It can process prompts, understand context, and execute tasks that require multiple steps.
The model’s advanced reasoning allows it to handle structured automation with long-context memory, making it effective for decisions that depend on earlier inputs.
This capability improves the efficiency of automation workflows by dynamically generating relevant search queries, adjusting to varying task requirements, and managing more complex sequences of actions.
Why BQL Complements DeepSeek
BQL enhances automation by handling the technical challenges of web scraping and data extraction. It can access data behind login pages, bypass Cloudflare protections, and interact with sites that use advanced bot detection.
By capturing hidden API responses, including those sent through JavaScript XHR and fetch requests, BQL retrieves data that traditional scraping methods often miss. It also manages CAPTCHAs and maintains session persistence, allowing long-running tasks to continue uninterrupted. With DeepSeek, BQL provides the tools to extract and process data across web environments.
Scraping at scale requires more than just extracting data relevant to search terms; it requires a reliable extraction process. DeepSeek generates intelligent search queries, while BQL handles the scraping, even on sites protected by bot detection systems. This combination reduces manual input, improves data quality, and adapts to changing market trends.
Let's see it in action in the example below. We will use DeepSeek, served through Ollama, to generate laptop-related search terms, validate and clean those queries, and then use BQL to scrape detailed product information (titles, prices, descriptions) from eBay.
Deployment Options
This setup is flexible and can be deployed in different environments based on your needs:
- Locally: Best for development and small-scale scraping tasks.
- In a Virtual Machine (VM): Provides resource isolation and controlled execution.
- In the Cloud: Ideal for large-scale scraping, automation, and handling long-running tasks.
Choosing the Right Model
The DeepSeek model you select depends on your available hardware:
- deepseek-r1:7b requires approximately 12GB RAM in CPU mode.
- Larger models (13b, 30b) improve query generation quality but demand more memory.
- Cloud-based execution is recommended for scaling beyond local hardware constraints.
Step 1: Generate a Search Query Using DeepSeek
DeepSeek generates precise search terms, removing the need to define queries manually. This function requests a short, targeted query related to gaming laptops in 2025.
What’s happening:
- Requests a concise search query related to gaming laptops in 2025.
- Parses the response to ensure only the search term is returned.
- Outputs a clean, usable search term for the next step.
Step 2: Scrape eBay Listings Using BQL
With the generated search term, BQL handles the scraping process. This function automates navigating to eBay, submitting the query, and extracting the results.
What’s happening:
- Navigates to eBay and waits for the page to load.
- Fills in the search box with the generated query.
- Submits the search form and waits for navigation to complete.
- Extracts the HTML of search results, which is passed to the next step.
Step 3: Extract Product Details from eBay Search Results
Once the HTML is retrieved, this function parses it to extract product details.
What’s happening:
- Loads the scraped eBay HTML into Cheerio for easy parsing.
- Extracts product details including title, price, and product link.
- Returns an array of product data ready for storage.
Step 4: Save Extracted Data to a CSV File
To store the extracted data, we write it to a CSV file.
What’s happening:
- Writes product data to a CSV file.
- Ensures valid URLs by appending
https://www.ebay.com
where needed. - Appends results to the file without overwriting existing data.
Step 5: Run the Full Scraper
This function ties everything together, executing each step sequentially.
What’s happening:
- Generates a search query using DeepSeek.
- Scrapes eBay results with BQL.
- Extracts product details using Cheerio.
- Saves the results to a CSV file.
Why This Is Better:
- DeepSeek generates intelligent search queries dynamically.
- BQL fetches & structures data from Cloudflare-protected sources.
- Works across multiple domains, unlike ChatGPT Operator’s API-restricted workflows.
Breaking Through Bot Detection: ChatGPT Operator vs. DeepSeek + BQL

Challenges with ChatGPT Operator
ChatGPT Operator struggles with advanced bot detection systems. It cannot modify TLS or JA3 fingerprints, making its requests easily identifiable. Without proxy support, all requests originate from static OpenAI servers, which increases the likelihood of detection.
Websites using Cloudflare’s JavaScript challenges or behavioral analysis often block these requests, limiting the operator’s ability to interact with protected content or complete multi-step web interactions.
DeepSeek + BQL’s Advantage
DeepSeek, combined with BQL, adapts dynamically to different websites and their protection mechanisms. DeepSeek uses AI to adjust request patterns, while BQL can spoof TLS fingerprints to resemble legitimate users.
BQL also handles real-time CAPTCHAs and Cloudflare challenges, allowing continuous access to protected pages. This combination improves the reliability of scraping workflows, even when dealing with sites that use strict bot detection techniques.
AI-Powered Large-Scale Scraping & Automation

Why ChatGPT Operator Falls Short
ChatGPT Operator has several limitations when handling large-scale scraping and automation. One limitation is that it restricts the number of API calls per request, which slows down data collection and makes scaling difficult.
As it lacks multi-threading capabilities, all actions are executed sequentially, which results in longer execution times for complex workflows. Additionally, many websites with advanced scraping protection can block their requests, limiting their ability to gather data from key sources.
DeepSeek + BQL’s Scalability
DeepSeek and BQL work together to handle large-scale scraping tasks more efficiently. BQL supports multi-threaded scraping, allowing it to process multiple websites in parallel, which speeds up data collection.
Persistent browser sessions reduce the need for repeated logins or re-authentication, making longer workflows more efficient. The ability to extract structured data across different platforms without being limited by API availability makes the combination of DeepSeek and BQL a more scalable solution for complex automation needs.
Conclusion
DeepSeek and BQL outperform ChatGPT Operator in web scraping and automation. DeepSeek generates relevant queries, while BQL extracts data from protected sites and efficiently handles large-scale tasks. Together, they enable adaptable, high-volume scraping without being limited by bot detection or API restrictions. Combining Browserless with DeepSeek offers an effective way to access structured data at scale for those looking to improve their data workflows. Sign up for a trial today.
FAQ
What makes DeepSeek and BQL better alternatives to ChatGPT Operator for web scraping?
DeepSeek and BQL offer greater flexibility and scalability than ChatGPT Operator. DeepSeek generates intelligent, context-aware search queries, while BQL handles advanced scraping challenges like bot detection, CAPTCHAs, and data extraction behind login pages. This combination enables large-scale scraping with multi-threading and persistent sessions, which ChatGPT Operator lacks.
Can DeepSeek and BQL bypass Cloudflare and other advanced bot detection systems?
Yes, BQL is designed to handle sophisticated bot detection methods, including those used by Cloudflare. It can spoof TLS/JA3 fingerprints, rotate user agents, and manage proxy usage to mimic human browsing behavior. With DeepSeek dynamically adjusting requests, the combination bypasses most bot protection mechanisms, allowing access to protected data.
How much RAM is required to run DeepSeek with Ollama for web scraping?
The hardware requirements depend on the DeepSeek model you choose. The deepseek-r1:7b model typically requires around 12GB of RAM when running in CPU mode. Larger models like the 13b
or 30b
versions require more memory but offer improved query generation quality. Consider deploying in a cloud environment or a VM with sufficient resources for large-scale scraping.
Can I use DeepSeek and BQL to extract large-scale product data from websites like eBay or Amazon?
DeepSeek and BQL are well-suited for extracting large volumes of product data from e-commerce platforms. DeepSeek generates targeted search queries, while BQL handles multi-threaded scraping, session persistence, and structured data extraction. This setup allows you to efficiently collect information like product titles, prices, and descriptions, even from sites with strict anti-scraping measures.